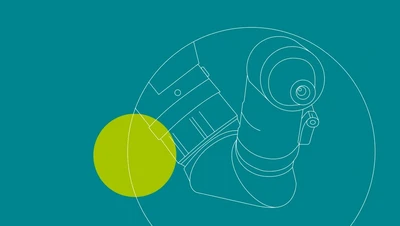
Automatic thinking
Apr 8, 2025
Can machines be as smart as humans? This question has been preoccupying the high-tech world for years. In the Dürr Group, too, dozens of experts are working on topics relating to artificial intelligence (AI). Searching for clues at the Bietigheim campus.
First stop: The treasure of the data lake
Today, Nico Koch has sat down at a bistro table. There are no more fixed workplaces in the Digital Factory. This also applies to the electrical engineer, who has been working with robotics and artificial intelligence for years. The head of department is currently working on a research project. Its aim is to drastically simplify the planning and design of paint booths. The project is part of the RoX research project funded by the Federal Ministry for Economic Affairs and Climate Action. In it, some 30 companies and research institutes are developing solutions in the field of AI robotics.
Today, when an automobile manufacturer puts out a tender for the construction of a paint shop, a team from Dürr spends weeks of planning in the first step. After the contract has been awarded, further weeks and months are required to work out the details of the project. “However, the times for planning and engineering could be drastically reduced,” says Koch. It sounds incredible, but it is possible — thanks to AI.
And this is how it could work: Dürr has been building paint booths for decades. All past projects are stored in the company as digital data. Although no two paint booths are the same, there are many common aspects. In the research project, algorithms are being developed that can analyze the huge amounts of data from past projects in a matter of minutes. From this, the program is to generate a plan for a paint booth that takes the customer’s needs into account.
Of course, long-standing employees can recall a lot of information from memory. That helps. “But even these specialists have by no means seen all the paint booths and are also becoming increasingly rare due to demographic developments,” says Koch.
Handing the job over to AI is not easy. After all, standards and norms have changed over the years. As a result, the data stored for the paint booths is often not comparable. “An important goal of the research project is to develop features that can be used to accurately select suitable data,” says Koch. This should not take too long — the RoX research project is scheduled to run for two and a half years.
Second stop: Intelligent hunt for errors
Jan-Philipp Schuh welcomes us in a small meeting room in the Digital Factory. The mechanical engineer is working on the further development of DXQplant.analytics — intelligent software that systematically detects quality defects in the production process and provides information on how to rectify them.
This is an important aspect for the painting process. Anyone who buys a new car attaches great importance to flawless paintwork. Manufacturers therefore inspect each car body for defects in a light tunnel at the end of the painting line. In modern paint shops, robot-guided camera systems are already used for this purpose. These digitally record the defects as accurately as possible. However, in many places this data still has to be analyzed manually, which takes a lot of time and requires immense expert knowledge. “This work can be automated with AI,” says Schuh.
The recorded quality defects are analyzed for recurring patterns using millions of stored data points. The software displays these quality defects in a virtual image of the car body.
“If defects occur frequently in the same place, it stands to reason that there is a common cause,” says Schuh. He tells of one manufacturer who tested a system after the occurrence of such a defect pattern and found the cause: Due to a defect in the area of a nozzle, it was constantly spraying liquid onto the paint. During the drying process, flaws then appeared on the painted surface. After the repair, the paintwork functioned perfectly again.
A flawless coat of paint saves car manufacturers a lot of money. Because every flaw has to be corrected by hand. For this reason, DXQplant.analytics is to get better and better. “In our vision, AI is the key to automated troubleshooting,” says Schuh.
Third stop: A chatbot that doesn’t spill the beans
Dominic Reiter welcomes us in a sitting area. In front of him are a notebook and a cup of coffee. That is all he needs for his work at the moment. As team leader, he is responsible for generative AI. This is a technology that independently generates new content, such as texts, images, or even videos, based on user requests.
Generative AI is intended to support Dürr Group employees in their day-to-day tasks. Basically, they could use the applications already available on the market, such as ChatGPT®. But that’s exactly what is not supposed to happen. “Anyone who uses such applications for free is paying with their data,” says Reiter.
The information of the company and its customers, however, shall remain protected. That is why Reiter and his team developed the company’s own Dürr AI chat. The digital assistant supports employees in a secure environment, be it searching for information, comparing documents or writing texts. Employees can also submit their requirements and wishes regarding the AI. As a result, the knowledge base of the Dürr AI chat is constantly growing, enabling it to assist with more and more use cases over time.
This is how an idea from the service department became a vision for Reiter and his team: Service employees usually search through huge amounts of technical documentation to find a solution to a technical problem. In the future, the digital assistant will sift through the vast amounts of data in a matter of seconds and display a suitable solution to the problem.
By the way, the exchange with the AI can be designed creatively, says Reiter, laughing: “The chatbot can not only answer in standard German, but also in the Swabian dialect.”
Machines that can think
Artificial intelligence — AI for short — is not a modern phenomenon. Let’s take a brief journey through some important evolutionary steps in machine learning.
1936
The foundation for AI is laid by the British mathematician Alan Turing as early as 1936. His theories prove that a calculating machine — the so-called Turing machine — would be capable of performing cognitive processes. This requires the processes to be broken down into several individual steps and represented by an algorithm.
1956
At a conference at Dartmouth College in New Hampshire (USA), scientists discuss how machines could simulate aspects of learning and other features of human intelligence. The term “artificial intelligence” is born.
1966
The first chatbot is created. The German-American computer scientist Joseph Weizenbaum invents a computer program that can communicate with people. He names the bot, which can simulate a human conversation partner, “ELIZA”.
1986
Thanks to the “NETtalk” program, the computer is given a voice for the first time and learns to speak. Example sentences and sounds are fed into the program, which is one of the early artificial neural networks. Building on this, it can draw its own conclusions: “NETtalk” can read words, pronounce them correctly, and apply what it has learned to new words.
2018
IBM's “Project Debater” engages in discussions with human debate champions on topics like space exploration or politics. And Google's AI “Duplex” can make a hair appointment or a restaurant reservation in a chatty tone over the phone — without the person on the other end realizing they’re speaking to a machine.
More about
- With Dürr's new manufacturing management software, customers can always design their production according to their preferences: → The building block principle
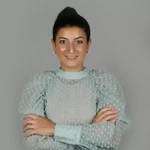
Tanja
Scheffel
Marketing
Dürr Systems AG
Carl-Benz-Straße 34
74321 Bietigheim-Bissingen
Germany
Carl-Benz-Straße 34
74321 Bietigheim-Bissingen
Germany